Inference Tagging - A Brief Overview
Inference Tagging goes beyond explicit concepts by deriving new information from implicit concepts in documents using existing datasets and specified rules. It leverages ontologies and rules to reveal hidden relationships, enabling interpretation, conclusions and predictions. This process is vital for automated decision-making, helping computing effectively understand complex data patterns and relationships.
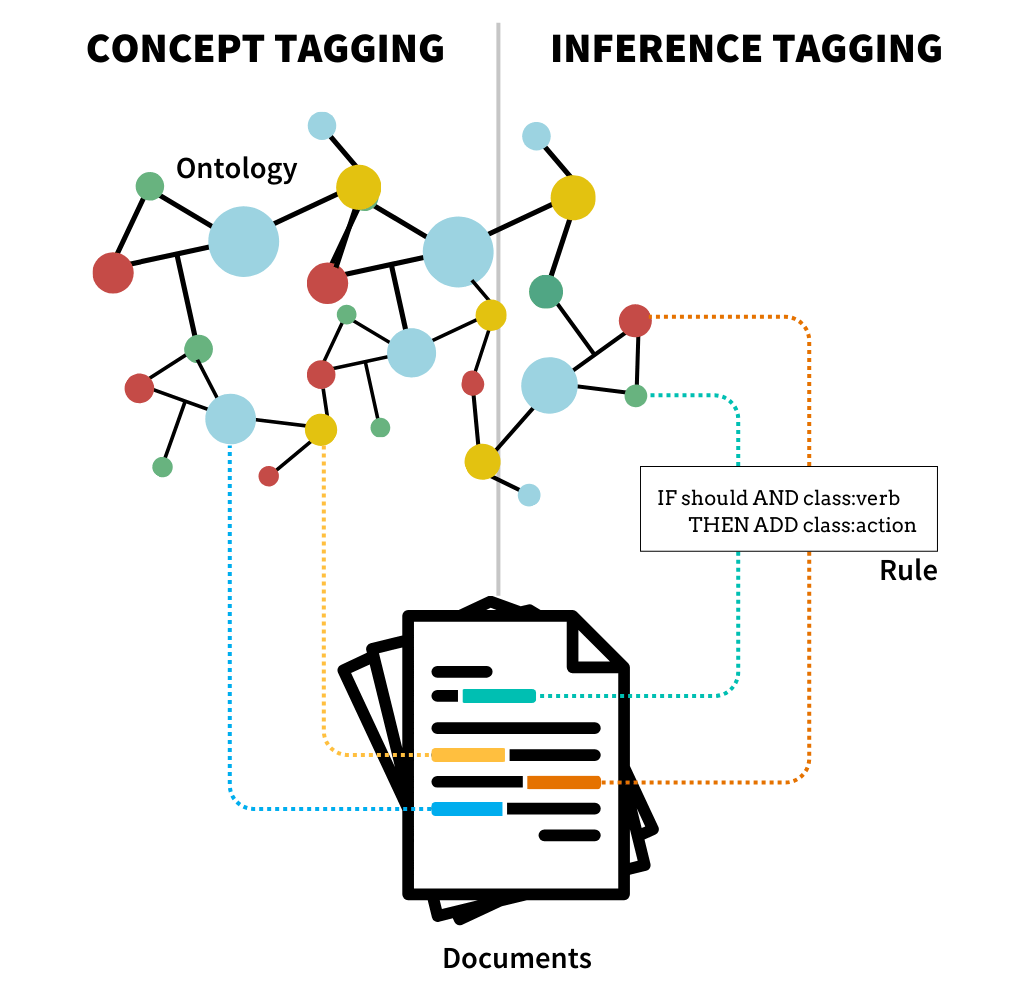
Inference Tagging is used in a wide range of applications including risk identification, pattern detection, personalized recommendations and decision support:
In healthcare, inference tagging of patient data can help to predict health risks, link to preventive measures or even indicate treatment interactions. In finance, it can ease impact assessments to infer between corporate measures and associated risks and opportunities.
With inference tagging, you are able to act on occurrence patterns of the concepts within documents or even their structural relationship. You can produce signals which can then trigger a certain classification of the document along with warnings, alarms or actions.
The flexible classification of documents according to changing readings or scenarios can be realized in modeling user behavior and user preferences in the inference rules enabling recommendation systems taking context, user intent and user behavior into account.
A document enriched with implicit concepts and facts using inference tagging makes it possible to link further background information already focused on the scope of the decision.